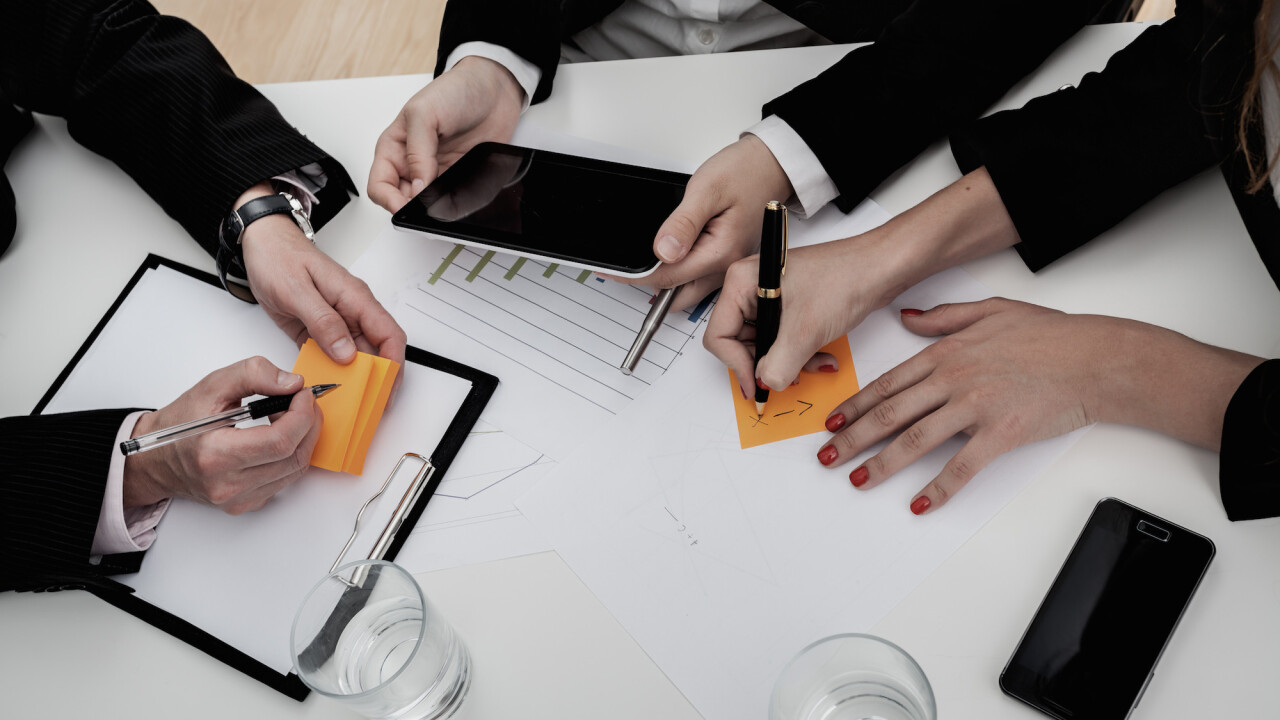
Jim Meyerle is the co-founder of Evolv, a big data company that optimizes workforce performance.
As a co-founder at a big data analytics software company, where recruiting talented data scientists and engineers often feels like finding a needle in a haystack, I regularly get asked about lessons learned in building world class, big data-focused R&D teams.
Given that the market for these applications has exploded in popularity, I believe it’s critical that companies in this space have a battle plan for how to recruit, develop and retain exceptional big data R&D talent.
We’ve focused on assembling an R&D team with overlapping capabilities. More than half of our 100-person staff are devoted to R&D and dozens more support our R&D efforts via a “leveraged network” approach.
While that includes engineers with requisite skills like Hadoop, the team also includes experts in other disciplines that have parallel (but unexpected) relevance to the work that we do: theoretical physics, artificial intelligence, organizational psychology, econometrics, and others.
Here are three practices I recommend:
1. Recruiting data science talent isn’t just about finding people with technical chops
Often, the more relevant (and overlooked) factor is where and how someone has been able to work with large, disparate datasets to solve broad business problems. To solve “thick market” problems, you want economists (economic consulting firms have troves of great people) to build the best probabilistic risk assessment models.
You’ll do great by hiring actuaries who code on the side, and many of the great minds in artificial intelligence built their core foundational knowledge in studying theoretical physics. Many of these disciplines are way more relevant to what a data scientist does on a daily basis than mastery of MapReduce libraries.
By identifying the industries and companies that have people with these types of backgrounds, you’ll have a major advantage.
2. A good number of the most hard-to-recruit big data talent is actually free
No, I’m not kidding. The best and brightest minds in big data science usually end up in a research role at a major academic research institution at some point in their lives.
With little-to-no practical work experience, Larry Page and Sergey Brin were certainly smart enough during their PhD program to solve one of the world’s most complicated data problems; Alex Karp (Founder/CEO of Palantir) did a PhD in Neoclassical Social Theory; and Joe Hellerstein (Founder/CEO of TriFacta) was a professor himself for a number of years before starting his own company.
What’s surprisingly unknown is that Masters or PhD candidates often have a hard time getting their hands on real-world, current datasets, and would jump at the chance of turning this data into insight as long as they can utilize those same findings to further their own research practice.
At Evolv, we’ve structured research partnerships with eight major U.S. universities (University of Pennsylvania and Northwestern among them), where Masters and PhD candidates (as well as tenured professors) work with our data to forward their research opportunities, while they also collaborate with us in developing incredible IP.
Working with a number of these talented minds also gives us a “first look” at some of the hottest candidates to hit the private job market – well before they’ve officially announced their candidacy.
3. The hardest-to-recruit top minds in the big data field are accessible if your company’s mission is linked to their own individual and personal interests
It’s shocking how little time founders spend in actually learning what the true interests are of the individuals they’re looking to recruit. Go to GitHub to figure out their engineering style, pay attention to their thesis. Several of our brightest technical minds across our R&D group joined us because our mission aligned with personal interests that they openly advocate.
Our chief analytics officer (PhD in Econometrics from Wharton) had always wanted to apply biostatistics methods to human capital data (he’s doing it now); our chief architect (doctoral studies in theoretical physics, MS computer science) felt that machine learning could more rapidly advance employer-to-employee matching outcomes, but hadn’t ever been able to find the right place to test his theory.
While there are plenty of other lessons learned in building big data R&D teams, the three above are the most important. They’re ultimately the tenets that have enabled us to develop big data products that scale, deliver huge value to our customers and their employees, and empower winning business models.
Get the TNW newsletter
Get the most important tech news in your inbox each week.