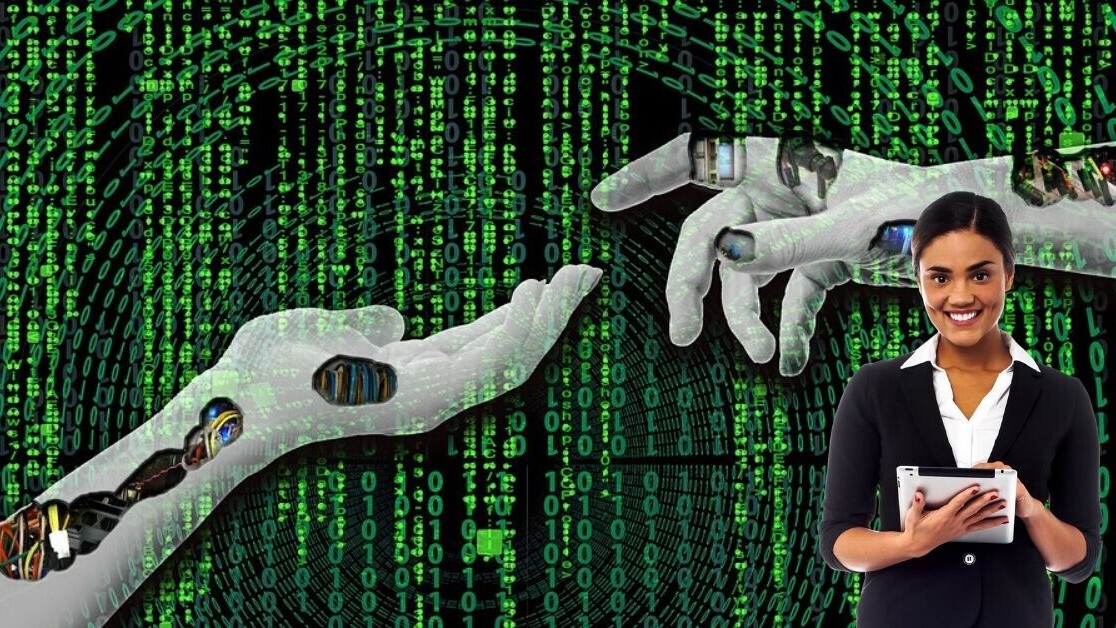
As AI, big data, and the internet of things (IoT) find their way into every aspect of our lives, many industries are undergoing a transformation. “Insurance executives believe that artificial intelligence (AI) will significantly transform their industry in the next three years, with insurers investing in AI to empower agents, brokers and employees to enhance the customer experience with automated personalized services, faster claims handling and individual risk-based underwriting processes,” consulting firm Accenture forecasted in 2017.
Three years later, AI algorithms have made great inroads in different sectors of the insurance industry and are lowering costs while driving efficiency and improving the customer experience. Slowly but surely, the industry is transforming. There are some kinks that need to be ironed out, but for the most part, the changes have been for the better, and there’s more down the road.
Here are three areas worth watching.
Big data and internet of things
Thanks to advances in edge hardware, cloud technology, and the internet of things, more and more information about objects, people, and organizations are being digitized. Telematics, wearables, and smart home sensors are just some of the technologies that are enabling us to collect detailed information about the physical world. And ubiquitous connectivity enables us to aggregate that data in cloud servers for further processing by machine learning algorithms.
In its 2018 Emerging Risk Report, Lloyd’s outlined some of the benefits that the growth of IoT will bring to the insurance industry, including better risk understanding, avoiding preventable losses, capturing patterns and behaviors and enabling proactive monitoring. Today, many insurers are embracing these trends to improve the speed and efficiency of their services. For one thing, having more data enables insurers to provide personalized and tailored premiums to individual customers.
One example is U.S.-based Layr, a cloud-based commercial insurance platform for small businesses, which came out of Lloyd’s insurance accelerator program, Lloyd’s Lab, and received funding in May to develop its AI-based solution. Layr uses machine learning to peruse customer data and compare applicants to clusters of similar businesses. This enables the company’s prediction engine to automatically match clients with the right policies.
Being able to collect rich, real-time data from the physical world through IoT sensors is also leaving its mark in the insurance industry. An example is Parsyl, an IoT startup that helps shippers, retailers, and insurers understand the quality conditions of sensitive and perishable products as they move through the supply chain.
Parsyl, another Lloyd’s Lab graduate, is incorporating its sensor technology in quality assurance and risk management solutions for clients that handle products that require specialist transport and storage. Such products included temperature-controlled foods, biological pharmaceuticals, and sensitive life science and high-tech products. Installing the sensors provides insurers with accurate data and insights about the cargo while giving the customers the benefit of expedited settlement, reduced claims cost, and machine learning–based risk mitigation.
One of the interesting trends in the field is the synergies between insurance and insurtech companies with specialized tech companies to enhance risk prediction and management. One interesting example is the partnership between weather forecasting company Climacell and Munich Re Syndicate, one of the leading marine and specialty underwriters at Lloyd’s. Climacell, which was part of Lloyd’s Lab’s third cohort, uses sensor data from various devices and machine learning algorithms to make precise weather forecasts. Munich Re Syndicate will use Climacell’s technology to help its clients better understand how the weather will affect their business and make informed decisions that enhance operational efficiency, safety and profitability.
In the car insurance sector, insurers use telematics to collect real-time driving data from vehicles. As opposed to the past, where they had to rely on basic information about the vehicle and driver to craft their insurance policies, they can now analyze telematics data with machine learning algorithms to create personalized risk profiles for drivers. Many insurers use this data to give discounts to drivers who have safe driving habits and penalize dangerous behavior such as speeding, hard braking, harsh acceleration, and hard cornering. The same data can help reconstruct accident scenes and enable insurers to better understand and assess what happened, which results in much faster claims processing.
In the health insurance sector, service providers use machine learning to help patients choose the best health insurance coverage options to fit their needs. Data collected from wearables such as fitness trackers and heart rate monitors help insurers monitor track and reward healthy habits such as regular exercise, and encourage preventive care by providing healthy nutrition tips.
An example is Insurtech startup Collective Health, which uses machine learning to identify risk and match its members with the right resources for their healthcare. The company’s AI model brings together claims data, prior authorizations, eligibility data, engagement data, and healthcare utilization data to develop a holistic profile of each member and their needs. The rich, AI-powered profile helps identify members’ health needs, such as those who need help from a pharmacist with confusing medications or need assistance from a nurse to help arrange home healthcare services.
“AI reduces the task of manually reviewing thousands of medical claims, and instead focuses our staff on performing warm, human outreach, and thinking through complex problems together with our members,” says Dr. Sanjay Basu, Collective Health’s Director of Research and Analytics.
One of the big advantages of the availability of data and machine learning algorithms is fraud prevention. Machine learning algorithms trained on the huge amount of data available on customers can glean patterns that separate legitimate claims from fraudulent ones. Today, most insurers use machine learning to detect and prevent fraud.
And with IoT and sensor technology continuing to expand at an accelerating pace, and with the propagation of 5G networks, we will continue to have even bigger datasets and accurate data to better understand insurance risks.
“I think that is one of the most exciting developments to come in the next decade is that we will have this incredible depth of knowledge about the world around us,” says Trevor Maynard, Head of Innovation at Lloyd’s.
Natural language processing
Computers have historically struggled to deal with data that is not neatly arranged in tables with rows and columns. But unfortunately, most of our data is unstructured and lies the documents, chat logs, emails, and the textual data we generate in our day-to-day interactions. Natural language processing, the science of helping computers understand and draw value from unstructured text, is a hot area of research and has seen tremendous progress in recent years.
The insurance sector, which is laden with textual data, has benefitted immensely from advances in NLP. Insurers have been able to leverage language models to reduce the time it takes to respond to customer queries and find relevant information from the tons of documents they must review in claims settlement.
An example is Lloyd’s International Trading Advice (LITA), a consultancy within Lloyd’s that gives insurance companies regulatory information about the countries in which it operates. LITA covers more than 200 geographies, and the regulatory rules of each area is registered in lots unstructured documents.
Previously, LITA’s experts had to manually go through these documents to answer questions about regulations and compliance, which usually took several days per query. To optimize the process, the LITA team used the ton of data they had gathered through their interactions with their customers to train a question-answering AI model. They were able to develop a system that automated a large part of the consultation process and improved the service-level agreement from five days to less than an hour.
“The AI augments the role of the employees,” says Craig Civil, Head of Data Innovation, R&D and Analytics at Lloyd’s. “It makes their job far more satisfying because we automate 80% of the work, and the 20% are truly interesting one-off questions that you do need an experienced team that can do the research and answer.”
Advances in NLP have also ushered in a breed of customer service chatbots in different sectors, including the insurance industry. Established insurance firms such as Geico as well as insurtech startups such as Lemonade are using AI-powered chatbots to settle claims. These chatbots handle the low-level customer queries and free agents to handle more complicated tasks.
AI researchers continue to develop larger and more complicated models that can tackle more complicated language-related tasks. In the past year, we’ve seen the release of state-of-the-art language models such as OpenAI’s GPT-2 and Google’s Meena. While we’re still pretty far from developing AI that can truly understand human language, practical uses will emerge from continued advances in natural language processing. AI will do the legwork, gathering import data and highlighting trends in text data, making it easier and less costly for insurers to piece that information together and address their clients’ needs.
Computer vision
Computer vision is the science of enabling machines to extract meaning and context from visual data. In the past few years, computer vision has been advancing by leaps and bounds thanks to convolutional neural networks, AI models that can perform image recognition and classification tasks with stunning accuracy.
Insurers now use image recognition algorithms to automate many of the tasks that previously required human labor. Insurance firm Liberty Mutual uses AI to provide fast damage assessment of vehicle damage and claims settlement. Users take a picture of the damaged car with their smartphone and submit it to the AI Auto Damage Estimator, which uses a machine learning algorithm trained on thousands of car accident photos to assess damage and costs. The process doesn’t take more than a few seconds.
Computer vision is also enabling insurers to perform tasks that were previously impossible. An example is State Farm’s Drive Safe & Save platform, which uses AI to analyze in-car camera feeds and detect and provide feedback on unsafe behavior such as distracted driving and texting.
One of the interesting trends to watch in computer vision is advances in edge computing and edge AI. Computer vision tasks previously required applications to send their data to cloud servers, where AI algorithms leveraged massive compute resources to process and analyze the data. But in recent years, specialized hardware and more efficient machine algorithms are gradually enabling on-device AI inference. The improved speed and efficiency are paving the way for real-time analysis of visual data and risk assessment.
Despite its many exciting applications, AI-based insurance is still in its early stages and the best is yet to come. As technology continues to permeate our lives, AI algorithms will be able to provide faster and more accurate solutions, making the insurance industry much more pleasing and less frustrating for both clients and agents.
Get the TNW newsletter
Get the most important tech news in your inbox each week.