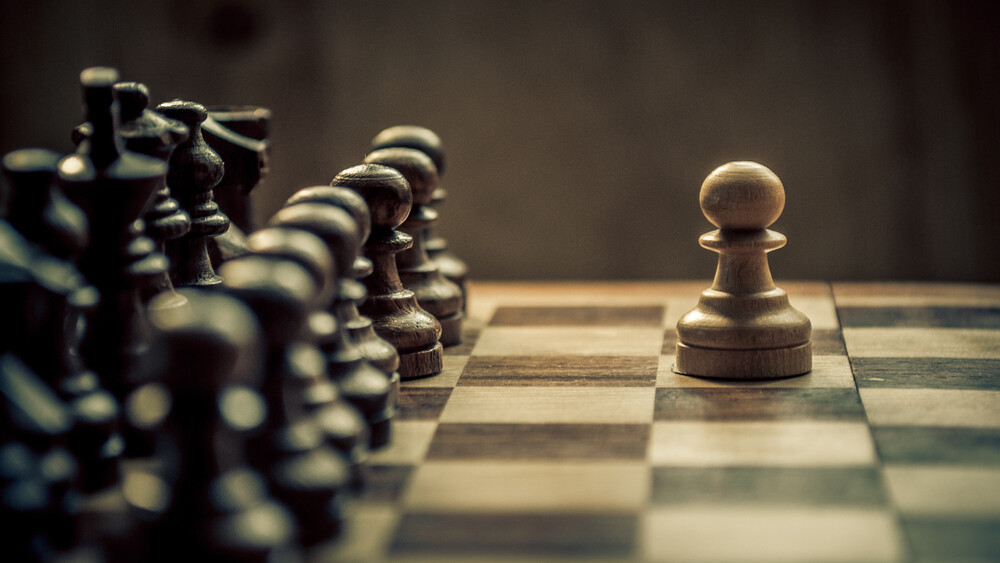
The Artificial Intelligence (AI) revolution was ignited more than half a century ago. In the last decade, AI has grown from an academic scientific field to start being a practical part of our everyday lives. The most common AI business strategies we see are built around data. We believe that proprietary data is currently the most strategic moat for AI companies, but in the coming years, it will become less of a unique asset, making proprietary data differentiation less sustainable. Therefore, we expect a shift in focus, from data-based AI strategies, to knowledge-based AI strategies.
The big data advancement, facilitated by the deployment of numerous sensors, internet connectivity and hardware and software improvement in computational power, communication abilities and digital storage, have enabled AI to scale from small academic research projects to large enterprise production applications. Essentially, big data required sophisticated AI models to analyze and derive knowledge and insights, while the AI models needed the critical mass of big data for training and optimization. Hence, at present, data is often perceived as a sufficient strategic moat for AI startups. As venture capital investors, we see this phenomenon routinely. In recent years, we have seen many startups that place data acquisition at the heart of their business strategy. An increasing number of such companies emphasize the unique data sets they have acquired and their long-term strategy for acquiring additional proprietary data – as a sustainable barrier of entry. Moreover, as AI tools and AI-as-a-service platforms have commoditized the development of AI models, and public data has become ubiquitous, the perceived need to build and defend a data moat has become palpable.
In today’s technology ecosystem, the markets have increasingly rewarded companies with leading AI programs and control over proprietary data – as a substantial and sustainable competitive advantage. Companies such as Google and Netflix have developed and curated massive and authoritative datasets over a long period of time, while many other companies struggled in vain to match their success. An example is the massive disruption of rival media service providers and production companies, which were outmaneuvered by Netflix’ sophisticated data strategy.
Nevertheless, due to expected advancements in the ability and willingness to exchange data, we believe that within a decade, proprietary data moats will be less sustainable. While data will still fuel the AI value engine, AI business strategies will be increasingly focused on knowledge.
Moving Up the AI Value Pyramid, towards the Knowledge Layer
The AI value pyramid is based on data and driven by knowledge. While today “we are drowning in information but starved for knowledge”, we expect moving up the AI value pyramid, towards the knowledge layer. Indeed, we have begun to see advances that will foster and accelerate this trend by creation of data exchanges. We expect that data exchange will be facilitated by a combination of increased feasibility and a willingness to share commoditized data in return for valuable knowledge. In summary, data will become more plentiful, available, reliable and standardized and inexpensive – the perfect definition of an ideal commodity. Using data as a sustainable barrier of entry will be more difficult in the future.
The increased feasibility to share data will be accelerated by the proliferation of data sources through the Internet of Things (IoT). In addition, there are new techniques, protocols and standards for pooling, sharing and exchanging data. Looking ahead, the increased ability to share data will become truly significant when there is incentive and a growing inclination to do so. As AI undermines and disrupts legacy competitive barriers to entry, many organizations relentlessly attempt to collect their own proprietary data and monetize it. Alas, this data acquisition and utilization is neither easy, nor fruitful and therefore creates strategic dissonance. This is because, while AI is increasingly indispensable for most organizations, it’s not part of their legacy skills or core expertise. In addition, the chronic and enduring shortage of engineers, developers, product leads and managers trained in AI sharpens this dissonance and leads to a solution preference for data sharing with the goal of knowledge exchange.
An example of the combination of ability and willingness creating through the exchange of data for knowledge generation is the new proposal by the European Union, to create “a single market for data,” in order to empower people, business and organizations to make better decisions based in insights from non-personal data in order to compete with the current tech giants.
Another factor contributing to data moats becoming less sustainable is the invention of novel data solutions which enable using smaller sets of data for training models. Synthetic data solutions (for example, with Generative Adversarial Networks) and other minimization techniques, like data augmentation, might allow companies to create disruptive AI products, without huge amounts of data.
Building a Knowledge Strategy
The future of the AI revolution will usher in a new reality for businesses and will require revised business strategy. The shift from data to knowledge will generate novel frameworks, partnerships and business models, encompassing different players bringing data, information, AI models, storage, and computing power, for knowledge creation. Since traditional data moats will become less sustainable in the coming decade, and knowledge will become the real value driver of AI, we believe businesses should begin crafting a strategy that is more focused on knowledge:
- Building knowledge moats, rather than data moats, is a fundamental principle that should be at the heart of future business strategy. Companies and organizations should start preparing for a knowledge-centric era, in which the winners will be those asking the right questions, looking for the most relevant predictions and designing the most disruptive AI-based applications.
- Use AI in a top-down manner and structure businesses around the application and product layer. Models should be developed and trained based on the specific vertical and hypothesis. For instance, developing specific health care applications in imaging, diagnostics, telemedicine, pharmacology and other clinical applications; or in mobility across fleet management, public transportations, and beyond. The development of these solutions will be based on deep knowledge and practical experience in specific domains, combining contextual knowledge and appropriate and well-tuned models.
- Data acquisition initiatives should be viewed as merely a short-term tactical pursuit while knowledge-based partnerships for exchange and cooperation should be fostered and cultivated as long-term business strategies. A productive example is that last year, the Israeli Innovation Authority launched a pilot program for knowledge-based cooperation between hospitals and technology startups. This cooperation generated dozens of initiatives[12] between hospitals of startups and facilitated the exchange of raw (and mostly unused) data from and among the hospitals, and generation of novel and valuable knowledge generated by the startups.
- Lastly, the shift towards knowledge should shape organizations’ HR strategy as well. Companies should develop a relevant and smart HR strategy for the future of AI. While some startups will still require hiring large cohorts of rare and expensive data engineers and scientists, savvy companies’ AI teams should instead be designed as a managerial group designed to pursue and foster AI knowledge partnerships, invent AI-based applications and products and creatively explore the bright horizons of the AI revolution – reimagined from data-centric to knowledge-centric. Furthermore, AI teams should have people who understand the context of the domain they are operating in. These contextual team members should encompass an holistic approach, originated in their understanding of AI and the specific domain, and not only general AI experts.
Overall, the future of AI depends on shifting from emphasis on proprietary data sets, to the sharing of data across entities for knowledge creation. To implement a successful AI strategy, companies must rightly combine data, information, AI models, storage, computing power and more, in order to root their business in knowledge.
About the Authors
Eviatar Matania is a professor at the School of Political Sciences, Government and International Affairs at Tel-Aviv University, where he heads the MA program of Security Studies and the MA program of cyber politics and government (under inception). Matania is also an Adjunct Professor at Oxford’s Blavatnik School of Government. Matania was the founding head and former Director General of the Israel National Cyber Directorate (INCD) reporting directly to the Israeli Prime Minister, and during the last two years he has been co-heading the Smart Systems (AI) National Initiative to recommend the Prime Minister and government about a national plan to posture Israel as a global power in AI.
Tomer Y. Avni is an associate at Blumberg Capital, based in Tel Aviv. Tomer connects with entrepreneurs, researches potential new investments, supports portfolio companies and develops emerging investment roadmaps. Prior to joining Blumberg Capital, Tomer served as a team leader and deputy group manager in the Intelligence Corps of the Israeli Defense Forces. During his tenure, Tomer was awarded excellence awards and led numerous technological research projects and operations. Tomer also worked as a tech consultant with a focus on cybersecurity. Tomer earned his B.Sc. in mathematics and political science, summa cum laude, from Bar Ilan University, at the age of 19.
We would like to thank David J. Blumberg, Jacob Rimer and Ori Rozen for contributing to this article.
Get the TNW newsletter
Get the most important tech news in your inbox each week.