There are plenty of examples of AI models being fooled out there. From Google’s AI to detect images mistaking a turtle for a gun to Jigsaw’s AI to score toxic comments tricked to think a sentence is positive by including words like love.
Now, researchers at Computer Science and Artificial Intelligence Laboratory (CSAIL), MIT, have developed a new system called TextFooler that can trick AI models that use natural language processing (NLP) — like the ones used by Siri and Alexa. This is important to catch spam or respond to offensive language.
TextFooler is a type of adversarial system that is often designed to attack these NLP models to understand their flaws. To do that, it alters an input sentence by changing some words without changing its meaning or screwing up grammar. After that, it attacks an NLP model to check how it handles the altered input text classification and entailment (the relationship between parts of the text in a sentence).
Altering text without changing its meaning is hard. First, TextFooler looks for important words that carry heavy ranking weightage for a particular NLP model. And then it looks for synonyms that fits the sentence perfectly.
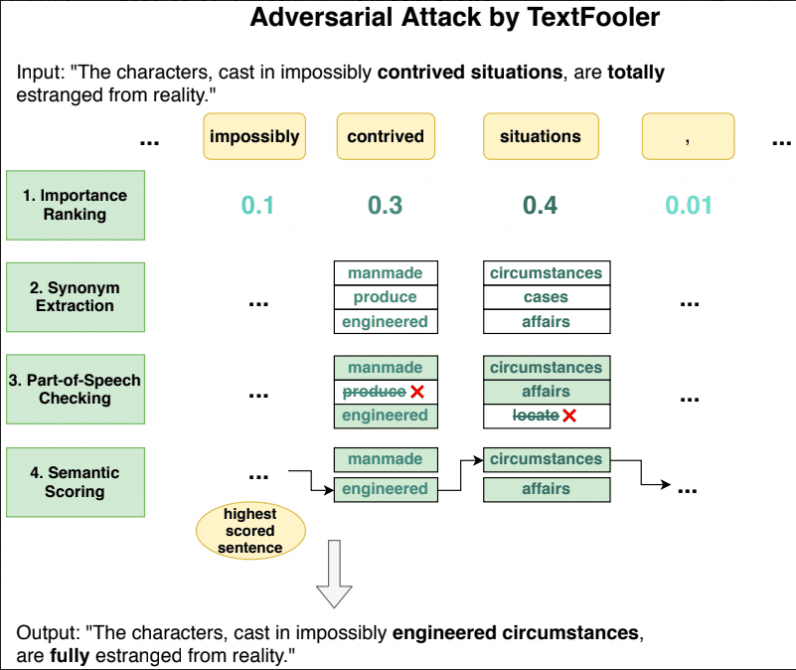
Researchers said that the system successfully fooled three existing models including the popular open-sourced language model called BERT, which is developed by folks at Google. By changing only 10 percent of the text in a sentence, TextFooler achieved high levels of success.
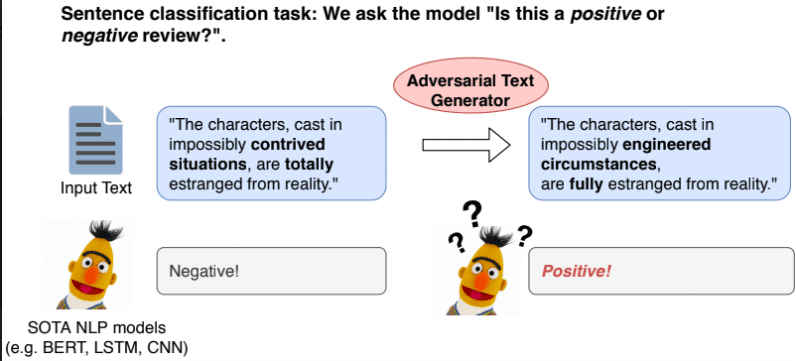
Di Jin, the lead author on a new paper about TextFooler, said that important tools based on NLP should have effective defense approaches to protect them from manipulated inputs:
If those tools are vulnerable to purposeful adversarial attacking, then the consequences may be disastrous. These tools need to have effective defense approaches to protect themselves, and in order to make such a safe defense system, we need to first examine the adversarial method
MIT’s team hopes that TextFooler can be used for text-based models in the areas of email spam filtering, hate speech flagging, or “sensitive” political speech text detection.
Google’s BERT is applied to the company’s search and many other products. And we often see that changing a few words in search can change results drastically. Even Alphabet-owned Jigsaw’s toxicity detection algorithm was tricked by changing spellings or inserting positive words into a sentence. This goes to show that it’ll take a lot more training to perfect language-based AI models before they can tackle complex tasks like moderating online forums.
Get the TNW newsletter
Get the most important tech news in your inbox each week.