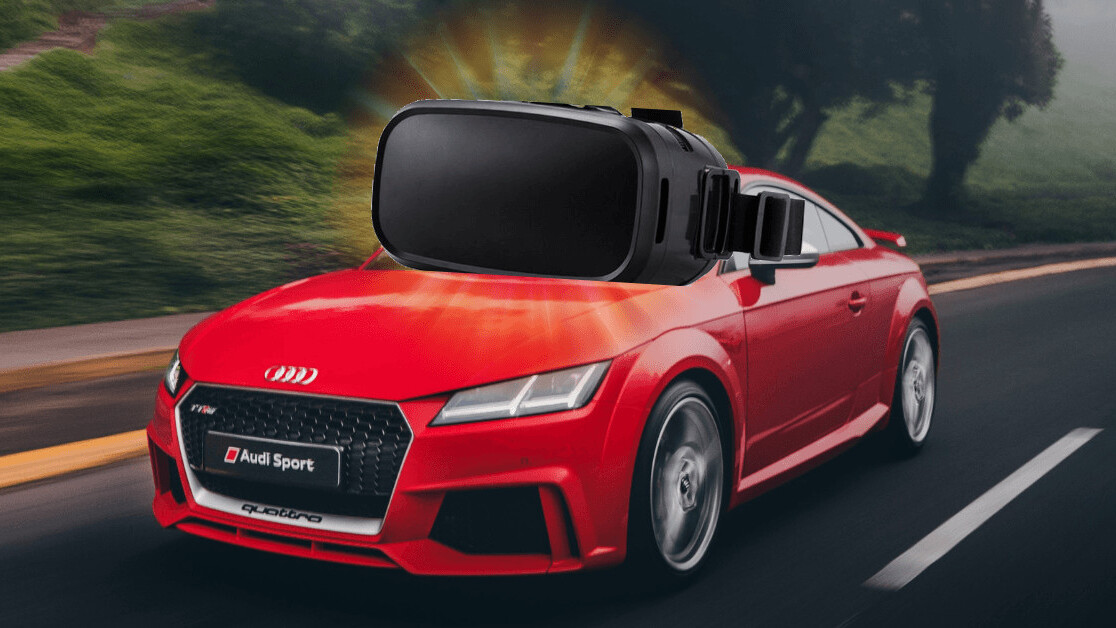
Twenty years ago, car makers were on the precipice of a major technological breakthrough. Until that point, systems such as braking and steering had been controlled by the mechanical certainty of gears, rods, and levers.
But by the late 1990s, manufacturers and key component suppliers started looking at replacing these physical systems with digital equivalents in the form of electronic motors and actuators. This was the first step in the development of what we’d now consider a modern digitally controlled car.
I was working in the automotive industry at the time, and I was surprised by how many among my colleagues, regulators, and society at large thought the new digital technologies would never be able to replace the certainty and predictability of mechanical systems.
Whenever a computerized component was incorporated into a system, they demanded it reflect a “physically re-creatable” process, as if a computer program could be structured exactly like a gearbox
They wanted computer code to work the same way that gears and levers had, because gears and levers were what they understood and trusted.
As a result, both automotive industry regulators and the average person on the street struggled to adapt their thinking and sense of security to a world where digital operations replaced mechanical certainty.
Inevitably, the many benefits derived from electronics triumphed in the end and the design paradigm for cars changed forever, along with our understanding of how to reckon with digital systems and accept their reliability.
Today the idea of building a new car without significant digital components is almost unimaginable. A pre-digital car’s lack of features, reduced efficiency, and lower safety standards would put off almost every potential buyer.
Fast forward two decades, and it feels to me like history is repeating itself as I watch friends, colleagues, and society at large react to machine learning (ML). ML delivers the ability for devices to teach themselves, adapt their behavior and start to become “artificially intelligent.”
Those who don’t understand ML or artificial intelligence (AI) can often react with a desire to hold on to the status quo. Like my former auto-industry colleagues, they want to bend new technology to be more like the systems they know – a desire that remains as misguided as it was in the 1990s.
But thanks to ML, we now have systems that are becoming statistically more accurate, more fluidly adaptable, and far superior in their ability to deal with complex situations than any computing system humans have ever had access to before.
However, these new systems have a new set of reasoning, fundamental assumptions, and even weaknesses associated with them, which many people struggle to fully grasp.
We are used to seeing our electronic systems as deterministic, reproducible, and “certain” – a world where A+B always equals C. It’s not a framework that translates well to ML techniques, which give us huge benefits but also reduce certainty and introduce a certain degree of inherent “inaccuracy.”
Some critics warn that AI will introduce new risks as humans devolve control to less deterministic systems and the corporations that control them.
The problem with the negative side of the AI argument is many people don’t understand how ML models differ from the conventional computer programs they have become comfortable with.
As ML is still emergent, this is not surprising, and questioning any new technology is always sensible. The problem is this questioning can also lead to a mindset like the one adopted by my former auto-industry colleagues—particularly the ones who thought that computer-based systems could never replace (and certainly not improve on) physical ones.
In order for ML and AI to advance, we must adopt a more pioneering mindset. We must evolve as humans to think about intelligent devices with a fresh perspective.
AI as black box
We can already see how governments are reacting to public concerns. The EU’s GDPR regulation that went into effect this month can be interpreted as recognizing a “right to explanation” for all citizens affected by decisions made by ML algorithms.
The rule (and the idea of explainable AI in general) is a reaction against the “black box” nature of ML algorithms: Because an algorithm teaches itself instead of following instructions written by human coders, it’s often difficult to tell why it makes its decisions, even when those decisions are more accurate than more traditional approaches.
Although a data scientist can adjust or tune an ML model so it’s less likely to yield a particular undesired outcome, they can’t simply go in and rewrite its code to eliminate the possibility of that outcome entirely, the way they could for a conventional computer program.
In practice, a right to explanation may place barriers in the way of realizing the new world of benefits ML and AI offer in important areas, from safety to health.
These types of social and legislative questions are arriving as existing AI systems are already improving human lives. Looking at the health sector alone, in the past few months the FDA approved AI that lets non-specialist doctors diagnose eye disease, and researchers released a free AI system that diagnoses 100 types of brain tumors more accurately than humans.
When AI systems’ recommendations are that accurate, requiring technologists to invent explanations for their decisions seems excessive.
There are many cases in which we trust an outcome without necessarily being able to fully explain it—for example, how many airline passengers understand the physical forces that lift their plane into the air? Boarding a flight requires a certain suspension of wisdom and an acceptance of an observed result. In short, trust.
The rise of fuzzy machines
We have the same trust issues with ML. Why is it so hard for most of us to trust the concept of a non-deterministic algorithm and wrap our heads around how it works?
In part, it is because we are used to computer programs that deal in exactitudes—given input X, they always return Y. ML models, however, deal in approximations and fuzziness, much like the human brain.
That means they can take in more varied inputs and make more sophisticated judgments than a conventional software program. But it also means that those judgements won’t be accurate 100 percent of the time (any more than a human’s ever would be).
Research shows that most people’s conception of how computers work hasn’t caught up to this notion of computer-as-fuzzy-approximator.
In a 2015 study, researchers from Wharton Business School found that people were even less likely to trust an ML algorithm after they had seen it make a mistake, even if that mistake was relatively small.
This was true even when the algorithm still outperformed humans at the assigned task. Interestingly, it seems that we humans still expect perfection from our machines, including when those machines are no longer designed to be perfect.
Great expectations
It’s worth noting that deep skepticism about ML may be mostly a Western problem. A 2017 survey by Northstar and Arm found that while only a little over one-half of Europeans and Americans expect AI to make society better, around three-quarters of people in Asia do. In China, that optimism has spurred a booming AI economy.
The Chinese government recently announced plans to become a world leader in AI by 2030, a plan it has backed up by making investments such as a $2.1 billion AI research park outside of Beijing and other initiatives.
As of this April, China is also home to the most highly valued AI startup in the world: Beijing-based SenseTime, whose most recent funding round gave it a valuation of $3 billion.
While there may be some potential advantages and disadvantages around the pace of deployment depending on where in the world ML and AI technologies are being worked on, there is one fundamental global truth.
The broad adoption of these advanced technologies requires a leap in the trust relationship between people and machines. We need to let go of the idea that a computer program must be exact and deterministic, and learn to accept approximation and fuzziness.
We need to stop trying to regulate machine learning models as if they were conventional computer programs, and start thinking about how to harness them for the betterment of society.
Get the TNW newsletter
Get the most important tech news in your inbox each week.