You’ve probably heard the phrase ‘deep learning’ bandied about in conversation, or maybe you’ve read about it in a post like this one. It seems like almost every tech conversation happening today somehow touches on the topic of AI, machine learning, or deep learning.
These technologies are coming into their own, and are poised to usher-in massive changes not only to the tech industry, but to every aspect of the global economy and society overall. But…what the heck does all of this mean?
In my position as a VC and as a managing director of scale-up program, I’ve worked with a number of startups that are leveraging deep learning along with other AI technologies. I recently created a white paper to help non AI experts understand the potential of deep learning to transform their business.
You can see the white paper here, but I wanted to highlight some of the ideas presented there while providing additional context about why deep learning is so important.
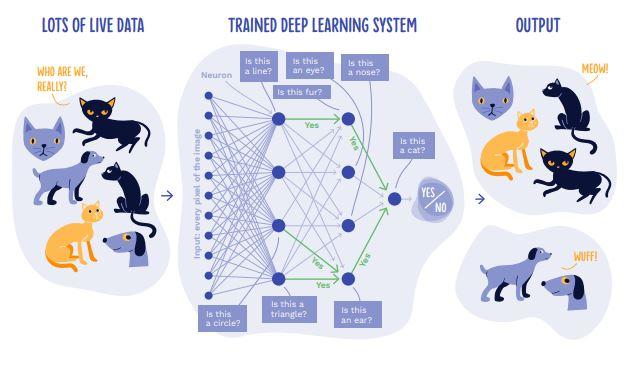
Why now?
There are key ingredients that are driving the development of deep learning: computing power, data, and AI as a Service.
AI has been around for a long time, tracing its roots all the way back to the 1950s. But there’s more computing power available today than ever before, and it’s starting to make powerful AI possible in ways that weren’t possible even a few years ago.
A lot of this is due to the computing power available via the cloud, but it can also be traced to the sheer advancements made in silicon. This is also the reason we are seeing all of the major players working on dedicated AI chips to take these advancements even further.
The key advancement that all this processing power has enabled is the ability to process the massive amounts of data needed to make AI… well, intelligent. AI is primarily about data and it’s being generated in staggering quantities. In just the last two years, 90 percent of all the data that has ever existed was created. In the world of AI, data is gold, it’s oil, it’s the valuable good that can’t be replaced.
What’s becoming more available and commoditized, however, are the actual AI algorithms needed to process all that data — a kind of ‘AI-as-a-Service.’
Many tech giants are offering AI algorithms via APIs through their cloud platforms. But no one can replace the data itself and it’s there that the hard work still has to be done. One example of that hard word is the labeling of data, which still has to be done by humans.
The reason is one way for machines to learn, is that they need to be fed accurate data that has been properly labeled and vetted. This is still a time consuming and expensive task, and it’s one of the reasons data is so valuable.
When looking at all three ingredients in total, it’s clear that data stands out as the clear winner in terms of value. AI-as-a-Service is a commodity available to all, computing power is a commodity available to all, but data is a valuable natural resource that emerges organically from technological platforms.
Like countries and natural resources, some platforms are data rich, and others data poor. Those companies that can leverage their data resources wisely stand to reap the greatest benefits of the AI revolution.
Machine learning and deep learning
“So what is this stuff already?” I hear you asking. Machine learning, of which deep learning is a subset, is the process by which machines are made more intelligent. Specifically, it’s a way for machines to learn without human interaction, or guidance. Machines are taught to recognize things, complete tasks, make predictions, and a number of other things.
Deep Learning is what I consider to be the “fanciest” of the different types of machine learning because it relies on neural nets, just like the human brain. Don’t be scared by that term — to understand a neural net just think of layers of functions built upon each other.
For example, imagine a scenario from daily life: you see an object, identify that it’s round, identify that it’s orange, and then identify that it’s a fruit, and therefore an orange. Each of those conclusions required a certain kind of function that recognizes a piece of the overall object and that feed off of the result of other functions.
So why is deep learning so fancy?
Now here comes the really exciting part. Deep learning is best suited for processing huge data sets. It enables several different ways of training machines that are truly exciting.
Most of the time, this is done through what’s called ‘supervised learning,’ which processes data that has already been labeled. However, there are other methods that hold incredible potential:
Unsupervised learning — With this method, you don’t tag the data. Rather you can simply throw in a massive amount of data, and task the system with finding patterns or clusters. This is very valuable in doing things like looking at user data to understand, which individuals are likely to convert into loyal customers.
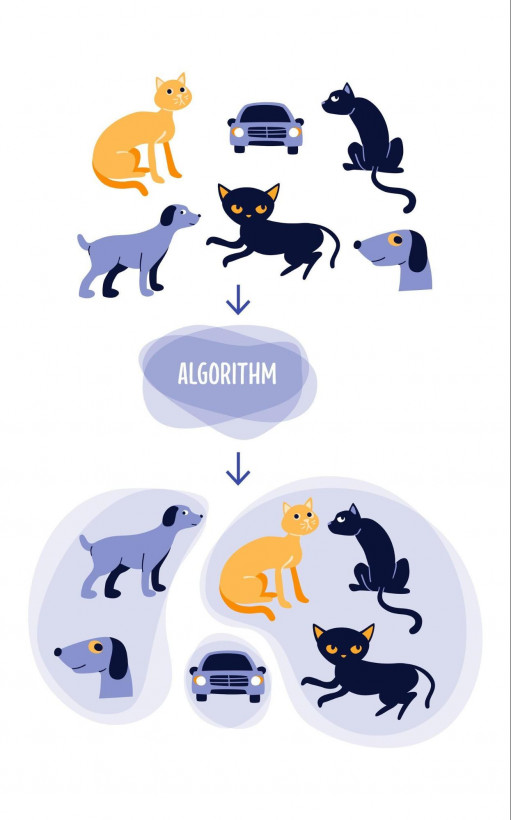
Reinforcement learning — Here it’s about training the system to achieve goals. The way it’s done is by giving the system a reward if it achieves something, and a penalty if it doesn’t. This method can be used for tasks like optimizing the starting position of an article on a page — a click is a reward for the system, and no click is a penalty.
Generative adversarial networks — This is a neural network architecture that features two AIs competing against each other with one AI trying to generate fake data, and the other to identify fake data.
For example, imagine an algorithm designed to generate fake videos of well-known individuals, such as celebrities or politicians pairing off against a counter algorithm designed to identify those fake videos. While this has the potential to create remarkably intelligent and creative AI, the potential for abuse is real, and the risks shouldn’t be dismissed.
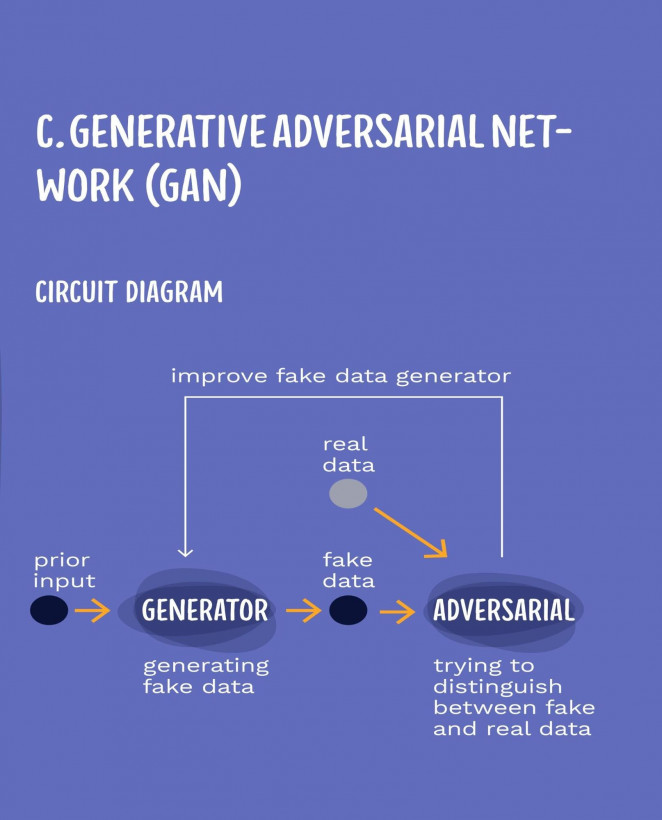
So what’s the bottom line?
As I said at the beginning, it feels like everyone is talking about AI right now. But why? A lot of the buzz is manifested as fear: fear that AI will take away our jobs; fear that AI will do us harm. There is some truth in those fears, especially when it comes to jobs.
AI does have the potential to take away jobs and eliminate some kinds of jobs altogether. Of course, AI will also generate new jobs, including roles that we can’t even imagine yet.
However, there is another, ironic, truth about AI: it will help us become more human. It will free us from the boring, monotonous tasks that are better suited for a machine. Doing that kind of work isn’t what we were made for.
Humans are imaginative, creative creatures. We are at our best when we are inventing new things, imbibed with emotion and beauty — not stuck in rubber stamped, mass produced repetitive tasks.
Of course, other obstacles remain. The data we have today isn’t clean enough, algorithms are still in their early stages, and we still need massive amounts of computing power to drive all of this deep learning. Yet, the potential that deep learning holds to enable real, powerful AI solutions fills with me with excitement.
Already today, AI is being used for things as mundane as making us better marketers to more meaningful applications such as scanning medical images to look for disease and in autonomous cars which will make road travel dramatically safer. It makes me feel like a kid to imagine the future we could usher in, and I hope that after reading this you are feeling that excitement as well.
Get the TNW newsletter
Get the most important tech news in your inbox each week.