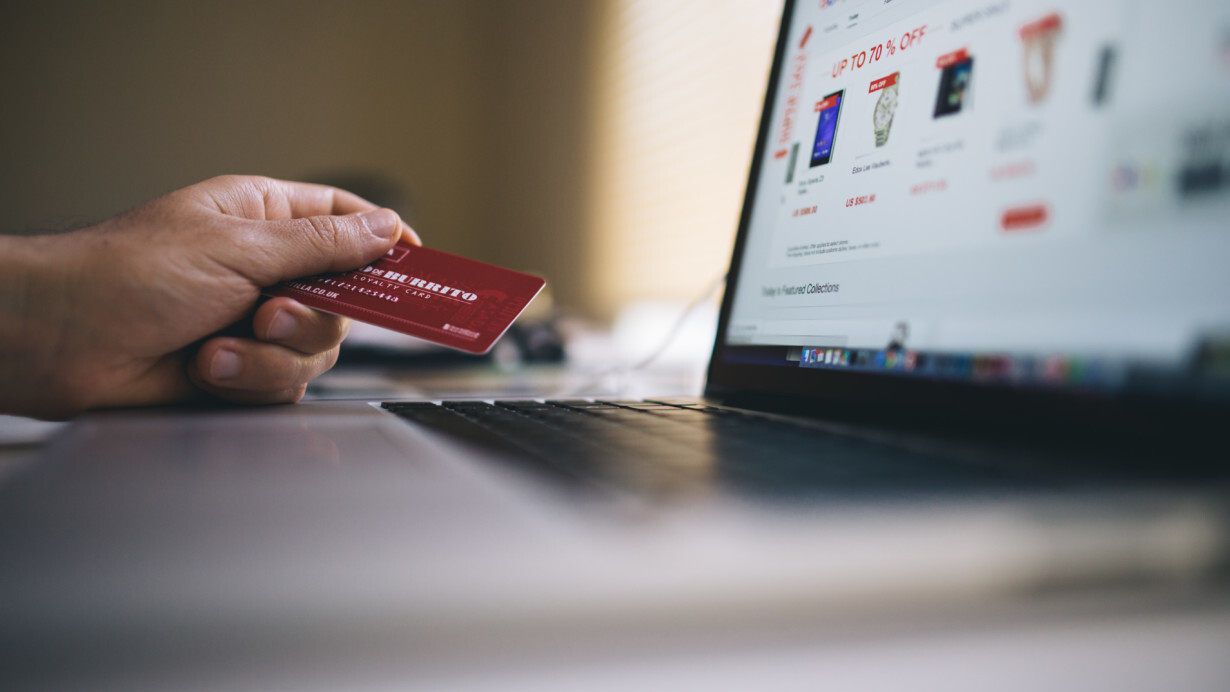
Remarketing seems deceptively simple. It’s a smart way for brands to reconnect with consumers who may have visited a website, but not necessarily pulled the trigger to make a purchase. It also allows you to position targeted ads to an audience who has previously expressed interest in products on your website, while they are simultaneously browsing the rest of the internet.
On top of that, it offers perfect targeting, where every single user can be lined up against a value proposition that needs to be delivered to them. Not only are the conversion payoffs high through this strategy, but the prevalence of second price auctions on exchanges ensures that both win rates and margins are healthy.
At first glance, it doesn’t seem like artificial intelligence (AI) or advanced machine learning (ML) techniques would have a major role to play here. Surprisingly, this is far from the reality.
Machine learning is a key ingredient involved in making remarketing effective. AI and ML solutions are often required when one encounters large scale, highly fragmented structures in very dynamic environments. This is especially true in the case of remarketing on both desktop and mobile. As such, there are four key ways in which AI and ML can be leveraged to make remarketing effective.
1) Needle in the haystack
The problem with perfect targeting is scale. If an advertiser has a named list of users, then it becomes imperative to find as many of them in all the places in the ecosystem where they might show up. In practical terms, this means having a seat at all the ad exchanges that could potentially get requests from mobile apps these users are running, and may involve looking up to tens of billions of ad requests every day.
Furthermore, this also means processing all that data on a regular basis. Not surprisingly, this can become very expensive, very quickly. Machine learning comes to the rescue in this situation by modelling where the targeted users are likely to appear. This reduces the number of requests that need to be examined, bringing it down from 100,000 requests-per-second, to a more manageable 1,000 requests-per-second. Typical techniques that can be effective here include look-alike modelling and running multivariate regressions to find dominant characteristics of the targeted group.
2) Seizing the moment
Often — and especially on mobile — there might be hundreds of opportunities in a day to display an ad to the user. Effective advertising is displaying the right ad in the context that a user is most likely to complete their purchase. Factoring where the user is in his/her engagement lifecycle, along with the particular spatio-temporal context can make a big difference.
For example, for one advertiser, we found that users are 20 percent more likely to click an ad within 100 meters of their home. This situation becomes tougher when there are multiple value propositions available to be shown to the user. Choosing the right value proposition is a well posed problem for machine learning. The figure below illustrates how this could play out for a single user.
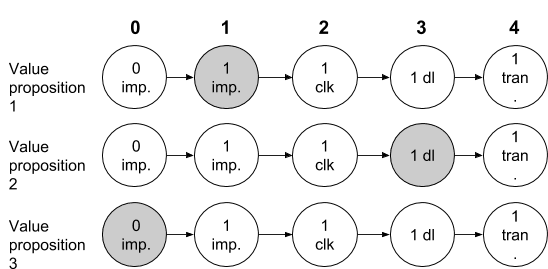
AI provides an ideal solution here by using data to build an understanding of the right value proposition for any context over hundreds of millions of instances and picking the right value proposition. According to a recent survey conducted by RocketFuel, more than 60 percent of millennials saw value in brands using AI to deliver personalized offers.
3) Picking the most appropriate creative
Creatives are the main element driving a user’s physical interaction. Choosing the right creative for the appropriate context can make a huge difference in conversion rates. Creative elements that could help ads convert better include design elements such as background color, call to action, and theme, along with modification in messaging and dynamic attributes.
New practical uses for AI have expanded recently, particularly in deep neural networks, and are extremely powerful in understanding images. Before these advancements, the only way to learn the effectiveness of an image would be to run A/B tests or test campaigns. Now, with the latest algorithms, it’s possible to predict the potential performance of a creative even before it has been seen by a single user — this could potentially save an advertiser 30-40 percent in costs.
In addition, AI can be used to test and construct the most effective creatives in real-time. This is not lost on leading agencies such as JWT and Saatchi & Saatchi that are testing out several AI driven creative processes.
4) Juggling a million balls
Formulating and maintaining the right campaign strategy — one that is highly customized, cognitive of the creative’s intrinsic life and the user’s history of interaction — can be a formidable task. This is another great application for AI. It can rebalance budgets, swap out poorly performing creatives, and change targeting on-the-fly to deliver on the objective of the campaign.
Remarketing at scale is fraught with challenges that involve dynamic complexity. AI methods can be gracefully deployed to surmount these challenges, allowing the marketer to focus on larger questions such as the objective of the campaign, and the desired impact.
Get the TNW newsletter
Get the most important tech news in your inbox each week.