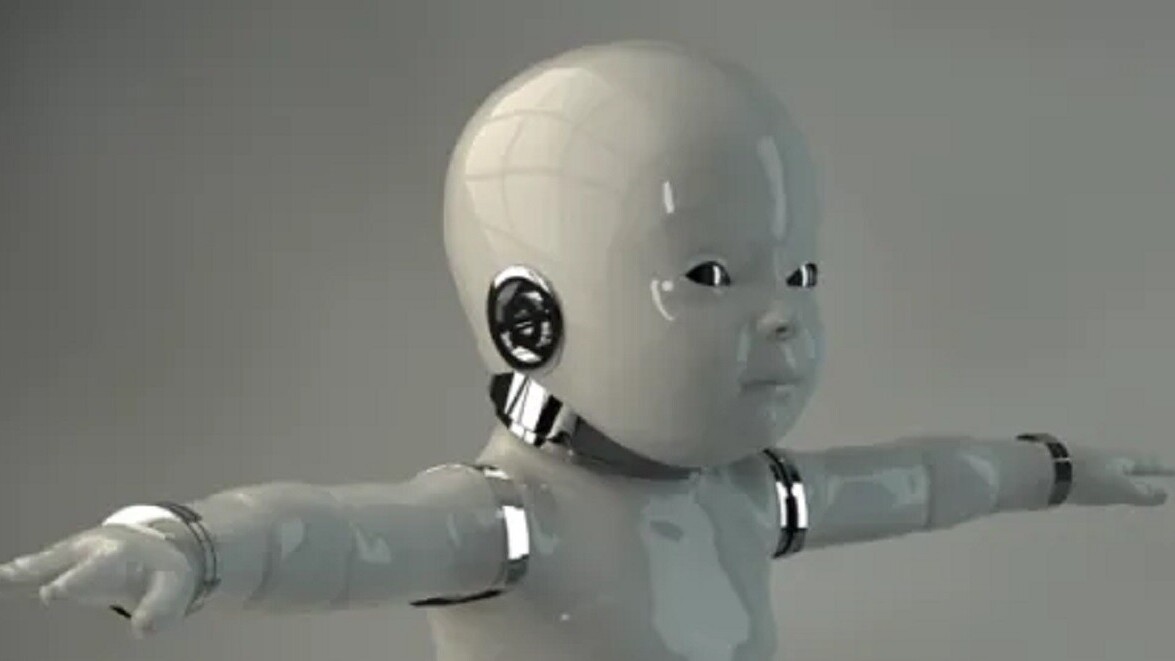
Artificial intelligence is, undeniably, one of the most important inventions in the history of humankind. It belongs on a fantasy ‘Mt. Rushmore of technologies’ alongside electricity, steam engines, and the internet. But, in it’s current incarnation, AI isn’t very smart.
In fact, even now in 2020, AI is still dumber than a baby. Most AI experts – those with boots on the ground in the researcher and developer communities – believe the path forward is through continued investment in status quo systems. Rome, as they say, wasn’t built in one day and human-level AI systems won’t be either.
But Gary Marcus, an AI and cognition expert and CEO of robotics company Robust.AI, says the problem is that we’re just scratching the surface of intelligence. His assertion is that Deep Learning – the paradigm most modern AI runs on – won’t get us anywhere near human-level intelligence without Deep Understanding.
In a recent article on The Gradient, Marcus wrote:
Current systems can regurgitate knowledge, but they can’t really understand in a developing story, who did what to whom, where, when, and why; they have no real sense of time, or place, or causality.
Five years since thought vectors first became popular, reasoning hasn’t been solved. Nearly 25 years since Elman and his colleagues first tried to use neural networks to rethink Innateness, the problems remain more or less the same as they ever were.
He’s specifically referring to GPT-2, the big bad text generator that made headlines earlier this year as one of the most advanced AI systems ever created. GPT-2 is a monumental feat in computer science and a testament to the power of AI… and it’s pretty stupid.
Read: This AI-powered text generator is the scariest thing I’ve ever seen — and you can try it
Marcus’ article goes to great lengths to point out that GPT-2 is very good at parsing incredibly large amounts of data while simultaneously being very bad at anything even remotely resembling a basic human understanding of the information. As with every AI system: GPT-2 does not understand anything about the words its been trained on and it does not understand anything about the words it spits out.
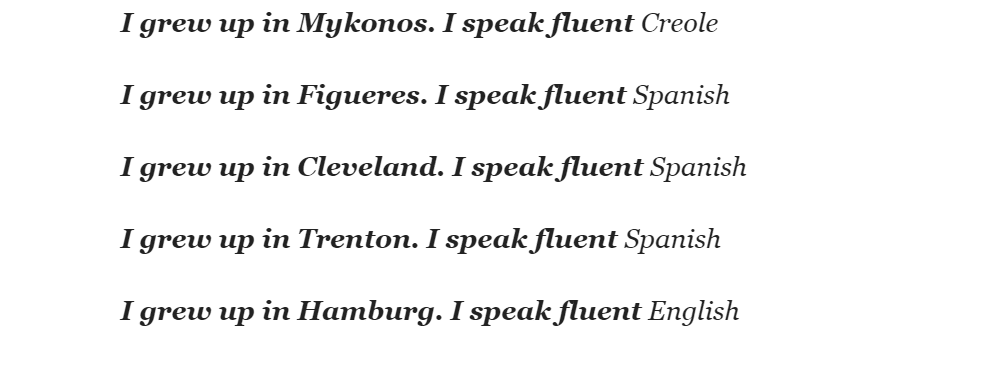
The way GPT-2 works is simple: You type in a prompt and a Transformer neural network that’s been trained on 42 gigabytes of data (essentially, the whole dang internet) with the ability to manipulate 1.5 billion parameters spits out more words. Because of the nature of GPT-2s training, it’s able to output sentences and paragraphs that appear to be written by a fluent native speaker.
But GPT-2 doesn’t understand words. It doesn’t pick specific words, phrases, or sentences for their veracity or meaning. It simply spits out blocks of meaningless text that usually appear grammatically correct by sheer virtue of its brute force (1.5 billion parameters). It could be endlessly useful as a tool to inspire works of art, but it has no value whatsoever as a source of information.
In the following image, Marcus gets some very bad advice from GPT-2 on how to handle ingesting hydrochloric acid:
GPT-2 is impressive, but not for the reasons many people might think. As Marcus puts it:
Simply being able to build a system that can digest internet-scale data is a feat in itself, and one that OpenAI, its developer, has excelled at.
But intelligence requires more than just associating bits of data with other bits of data. Marcus posits that we’ll need Deep Learning to acquire Deep Understanding if the field is to move forward. The current paradigm doesn’t support understanding, only prestidigitation: modern AI merely exploits datasets for patterns.
Deep Understanding, according to a presentation Marcus gave at NEURIPS this year, would require AI to be able to form a “mental” model of a situation based on the information given.
Think about the most advanced robots today: the most they can do is navigate without falling down or perform repetitive tasks like flipping burgers in a controlled environment. There’s no robot or AI system that could walk into a strange kitchen and prepare a cup of coffee.
A person who understands how coffee is made, however, could walk into just about any kitchen in the world and figure out how to make a cup, provided all the necessary components were available.
The path forward, according to Marcus, has to involve more than Deep Learning. He advocates for a hybrid approach that combines symbolic reasoning and other cognition methods with Deep Learning to create AI capable of deep understanding, as opposed to continuing to pour billions of dollars into squeezing more compute or larger paramater packages into the same old neural network architectures.
For more information check out “Rebooting AI” by Gary Marcus and Ernest Davis and read Marcus’ recent editorial on The Gradient here.
Get the TNW newsletter
Get the most important tech news in your inbox each week.