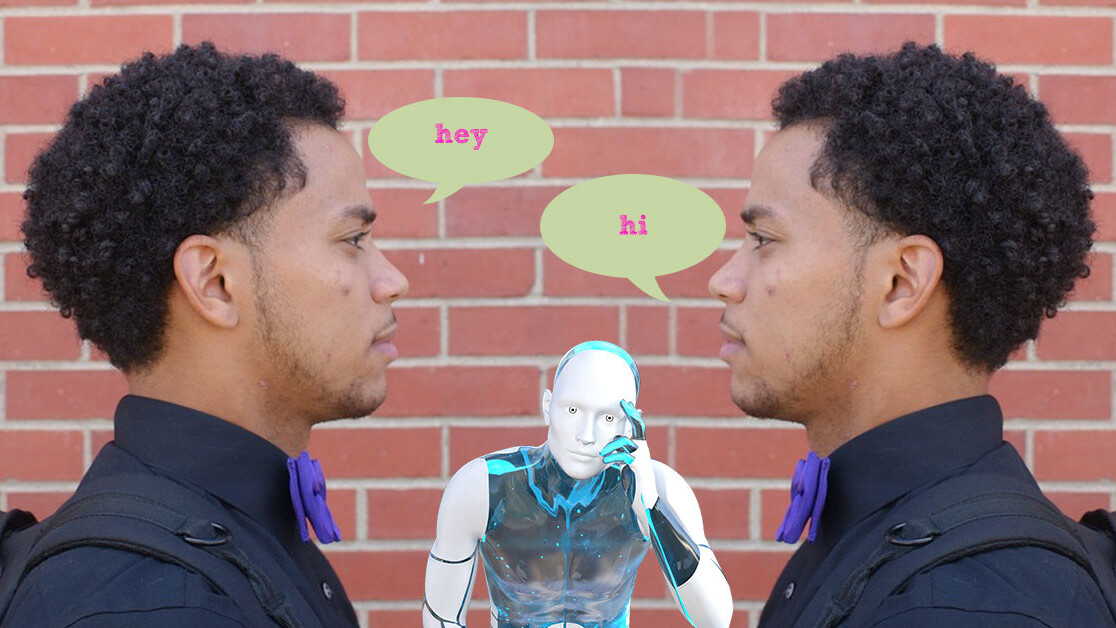
A team of researchers from the University of Edinburgh developed a novel method for teaching AI to respond to queries in a more conversational manner: They paid people to talk to themselves.
It turns out that paying one person to pretend to be two people is a cost-effective way of training an AI to recognize natural human language. That may seem like a no-brainer, but the interesting part is that it also produces better results than current multi-speaker conversation datasets.
Here’s an example from the self-dialogue database — despite the denotations this is a single study participant providing both sides of the conversation:
① What is your absolute favorite movie?
② I think Beauty and the Beast is my favorite.
① The new one?
② No, the cartoon. Something about it just feels
magical.
① It is my favorite Disney movie.
② What’s your favorite movie in general?
① I think my favorite is The Sound of Music.
② Really? Other than cartoons and stuff I can
never get into musicals.
① I love musicals. I really liked Phantom of the
Opera.
The researchers set out to improve the conversational quality of virtual assistants by streamlining the data gathering process. Current state-of-the art human conversational datasets are gathered through more traditional means, such as getting two people to talk on the phone, recording the conversation, and transcribing the audio for parsing by a neural network.
Unfortunately, this can result in a disconnect: When two random strangers speak there’s often a lack of general understanding which causes the conversations be stilted or produce less-than-desirable communiques. Furthermore, according to the researchers, it makes it difficult to find people willing to invest the time it takes to participate in these studies.
This study was conducted using Amazon’s Mechanical Turk, a crowdsourcing platform where workers complete tasks online for small amounts of money. These workers rely on their ability to quickly complete one task and move on to the next in order to earn money – Turk tasks typically only pay a few cents.
When two Workers have to cooperate on a project they have to wait for one another to respond, according to the researchers this stunts their work:
This led to a median time for a Worker to complete a HIT of roughly 14.9 minutes (average response time 37 seconds). This proved to be unbearable for some of the workers and as a result the percentage of complete HITs was only 50.80%. In contrast, the median completion time for the self-dialogues was 6.5 minutes.
So can one person actually hold a conversation with themselves that would be useful to a neural network? The answer is a definite yes. The study shows the self-dialogue dataset outperforms state-of-the-art datasets compiled through crowdsourcing two-party conversations or ripping them from available recorded sources.
Listed below are conversation samples generated using a dataset entitled Open Subtitles (OS) and the Edingburgh team’s Self-dialogue dataset (SD). The “Q” denotes the query the machine was given.
Q: hello
OS: what a surprise
SD: hi there
Q: how are you
OS: why is your pajama to on
SD: doing good and you
Q: what do you want to talk about
OS: why did you invite me to stay for the holidays
SD: let’s talk about football
Q: what is your favorite movie
OS: star wars of course
SD: oh that’s a tough one i think the prestige
Q: which harry potter movie did you like best
OS: good one goyle
SD: i loved all of them
Q: who will win the superbowl
OS: give me another magazine quick
SD: indianapolis colts
It’s obvious that the Open Subtitles database often produces unexpected results that either have no connection to the query, or appear to contain statements that allude to occluded information. The SD database, on the other hand, seems to uniformly contain answers that would be satisfactory in most circumstances.
Interestingly, looking at the above responses, there’s a bit of crossover with current virtual assistants. If you ask Google Assistant its favorite movie it says “Star Wars,” and Alexa say its is “The Empire Strikes Back.”
For better or worse, the current trend in natural language processing for virtual assistants is to have them respond “conversationally” like a human would. Which means we’re trudging through yet another Uncanny Valley until such a time as researchers can come up with a convincing chat bot.
Hopefully the development of more streamlined and robust methods for creating human language datasets for AI will lead to a better back-and-forth between us and the machines. Because right now a person could go hoarse trying to figure out how to say the right thing to get any information beyond the time and weather from a smart speaker.
Get the TNW newsletter
Get the most important tech news in your inbox each week.