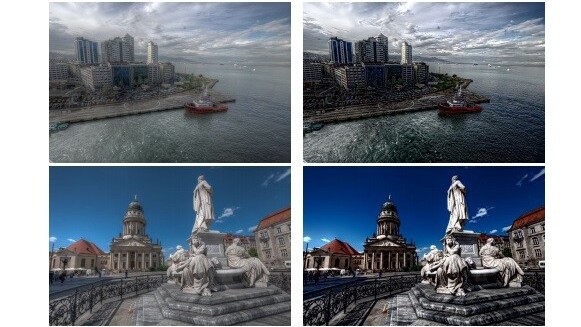
Google’s AI researchers recently showed off a new method for teaching computers to understand why some images are more aesthetically pleasing than others.
Traditionally, machines sort images using basic categorization – like determining whether an image does or does not contain a cat. The new research demonstrates that AI can now rate image quality, regardless of category.
The process, called neural image assessment (NIMA), uses deep learning to train a convolutional neural network (CNN) to predict ratings for images.
According to a white paper published by the researchers:
Our approach differs from others in that we predict the distribution of human opinion scores using a convolutional neural network … Our resulting network can be used to not only score images reliably and with high correlation to human perception, but also to assist with adaptation and optimization of photo editing/enhancement algorithms in a photographic pipeline.
The NIMA model eschews traditional approaches in favor a 10-point rating scale. A machine examines both the specific pixels of an image and its overall aesthetic. It then determines how likely any rating is to be chosen by a human. Basically, the AI tries to guess how much a person would like the picture.
This doesn’t bring us any closer to machines that can feel or think – but it might make computers better artists or curators. The process can, potentially, be used to find the best image in a batch.
If you’re the type of person who snaps 20 or 30 images at a time in order to ensure you’ve got the best one, this could save you a lot of space. Hypothetically, with the tap of a button, AI could go through all of the images in your storage and determine which ones were similar, then delete all but the best.
According to a recent post on the Google research blog, NIMA can also be used to optimize image settings in order to produce the perfect result:
We observed that the baseline aesthetic ratings can be improved by contrast adjustments directed by the NIMA score. Consequently, our model is able to guide a deep CNN filter to find aesthetically near-optimal settings of its parameters, such as brightness, highlights and shadows.
It might not seem revolutionary to create a neural network that’s almost as good at understanding image quality as humans are, but the applications for a computer with human-like sight are numerous.
In order for AI to perform tasks in the real world, like safely driving a car without human assistance, it has to be capable of “seeing” and understanding its environment. NIMA, and projects like it, are laying the groundwork for the fully-capable machines of the future.
Get the TNW newsletter
Get the most important tech news in your inbox each week.